Disparities in COVID-19 Incidence: A Simple Method for Imputation of Missing Race/Hispanic Ethnicity
- Dr. Beth Pathak
- Aug 12, 2022
- 7 min read
Updated: Aug 12, 2022
Précis:
In this post, I describe the problem of missing race/ethnicity information for COVID-19 cases reported to the U.S. Centers for Disease Control and Prevention (CDC) by state health departments. These missing data impair our collective ability to monitor racial/ethnic disparities in COVID-19 incidence. A simple method is proposed for imputing race/ethnicity for aggregate counts of cases – not for individual cases – on a state and age-specific basis. Imputing race/ethnicity for state-level COVID-19 case counts permits calculation of more accurate incidence rates, and evaluation of racial/ethnic disparities at the state and national level. The method is illustrated with real-world data for children and adolescents aged 0-19 years in the state of California. I conclude with a discussion of the limitations of this imputation method, and highlight ongoing gaps in U.S. public health surveillance of COVID-19.
Please cite this post as follows:
Pathak EB. “Disparities in COVID-19 Incidence: A Simple Method for Imputation of Missing Race/ Hispanic Ethnicity.” The COVKID Compass, Women’s Institute for Independent Social Enquiry, August 12, 2022. https://www.covkidproject.org/post/simple-method-race-ethnicity-imputation.
Background on COVID-19 Case Surveillance
In the United States, primary responsibility for surveillance of incident COVID-19 resides with state health departments, not the federal government (Figure 1). The federal government, specifically the CDC, passively receives new case (incidence) data from state governments, cleans and aggregates those data, and then analyzes and reports COVID-19 case data to a variety of audiences. It is important to note that while the CDC developed detailed COVID-19 data reporting guidelines for state health departments early in the pandemic and provides ongoing technical assistance, it does not have the legal authority to compel mandatory reporting. State reporting of COVID-19 cases is voluntary, as is all infectious disease reporting through the National Notifiable Diseases Surveillance System (NNDSS).
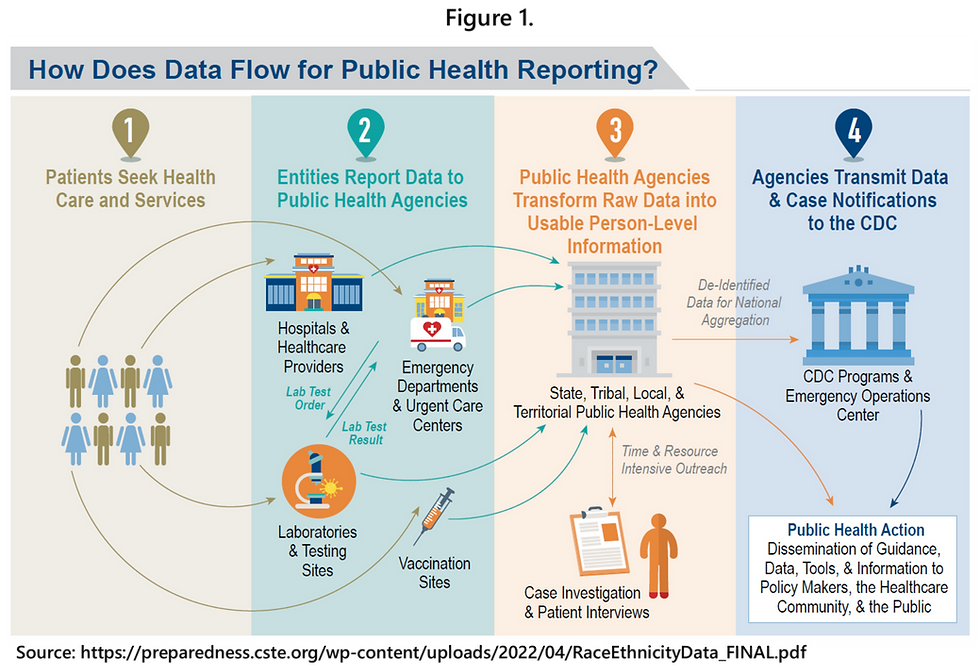
At the state level, new cases of COVID-19 are ascertained from clinical laboratories which conduct polymerase chain reaction (PCR) molecular tests of nasal swab samples of persons who are suspected cases. The PCR test directly detects the viral genetic material (ribonucleic acid or RNA) of the SARS-CoV-2 virus. COVID-19 PCR tests were first approved for use in the U.S. in February 2020, and a positive PCR test result is considered the gold standard (scientifically) for identifying cases and is also required to meet the official government definition of a confirmed case of COVID-19.
The first SARS-CoV-2 antigen test was approved by the FDA in May 2020. Rapid antigen tests for COVID-19 can be used to identify a probable case of COVID-19 only when the test is administered by a Clinical Laboratory Improvement Amendments (CLIA)-certified provider. Reporting of antigen test results by CLIA-certified providers to local and state public health agencies is required under the Coronavirus Aid, Relief, and Economic Security (CARES) Act (P.L. 116-136, § 18115). However, positive results on a home-use rapid antigen test cannot be used to officially define either a confirmed or probable case of COVID-19, despite the scientifically proven very high specificity of these tests (very high specificity of >99% means that the likelihood of false positive results is low). It is notable that the CDC webpage with the COVID-19 case definitions has not been reviewed since August 24, 2021. It still contains the criterion that more than 90 days must have passed from a previous infection before a person can be considered to have a new case of COVID-19 (i.e. reinfection).
The CDC maintains a public restricted-use caseline data file, updated monthly, which includes all COVID-19 cases reported by every state to the CDC. These data are de-identified and further anonymized through selective suppression of certain variables based on cell sizes of selected variable cross-tabulations. Demographic information reported for each case includes age group, gender, race/ethnicity, and county and state of residence. Age and gender data are more than 95% complete. However, there is a substantial degree of missingness for race/ethnicity of COVID-19 cases. Furthermore, the degree of missingness varies by state (Figure 2). For children and adolescents aged 0-19 years, the percent of cases in the CDC restricted-use file (April 4, 2022 release) who were missing race/ethnicity ranged from 9.2% in Utah to 100% in North Dakota, with more than one-third missing in the majority of states.
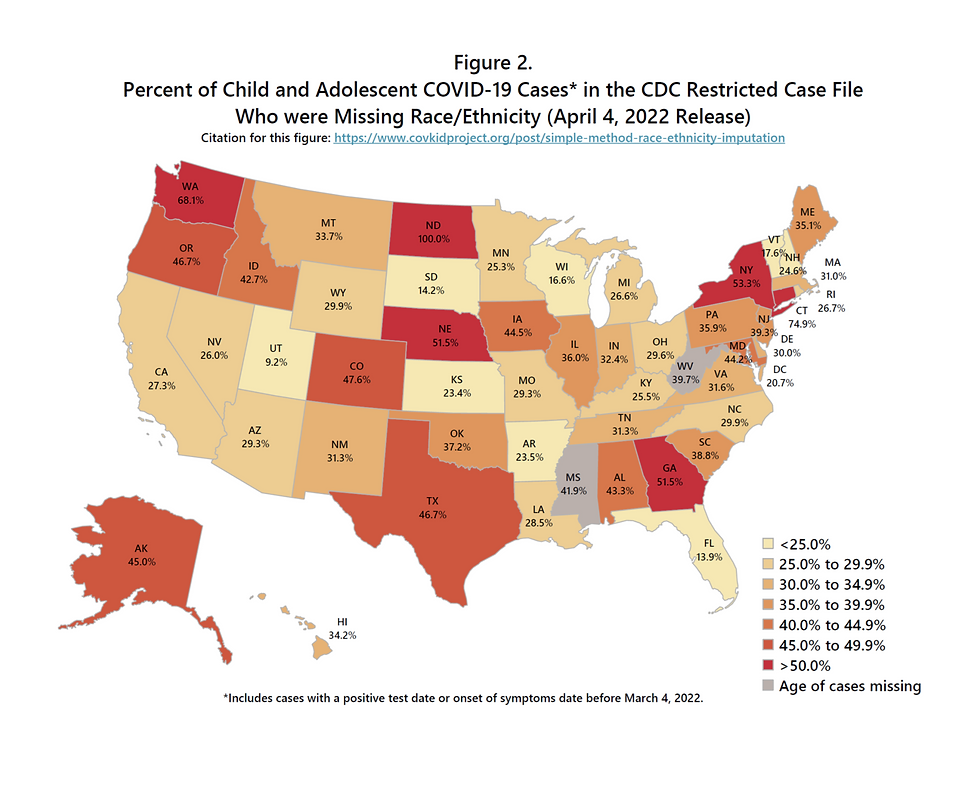
The CDC has acknowledged the problems with reporting of race/ethnicity and other detailed information about COVID-19 cases:
“The COVID-19 pandemic has strained the public health data supply chain. In many states, this has challenged hospitals, healthcare providers, and laboratories in reporting complete demographic information, such as race and ethnicity. The volume of cases has also made it challenging for state, local, and territorial health departments to conduct thorough investigations. As a result, some COVID-19 case notifications do not have complete information, even as health departments continue to make improvements through methods such as automated data flows. Missing data can affect interpretation of factors that might put people at higher risk for severe disease. Analyses of incomplete data elements are likely an underestimate of the true occurrence.”
In addition:
“Most of the case reports captured by health departments are based on laboratory reports that might contain limited patient information. Because of the volume of cases, most health departments are unable to obtain additional information on every case. As a result, many case reports are missing data on patient demographics, symptoms, underlying health conditions, characteristics of hospitalizations such as ventilator use, and other factors such as travel history. Because of missing data, analyses of these data elements are likely an underestimate of the true occurrence.”
Why are Race/Ethnicity Data Missing from COVID-19 Case Surveillance Data?
In May 2021, the Council of State and Territorial Epidemiologists (CSTE) undertook an investigation into the reasons behind missing race/ethnicity data, including various barriers at the local and state level. They surveyed state, tribal, and territorial health departments, along with a few large local health departments. Respondents were asked for their experiences and opinions about barriers to collecting and reporting race/ethnicity during routine COVID-19 surveillance. The majority of organizational respondents said that failure to report data by laboratories, limited public health agency resources and staffing for follow-up with cases, and patient hesitancy to self-report at the time of testing contributed to race/ethnicity data gaps (Table 1).
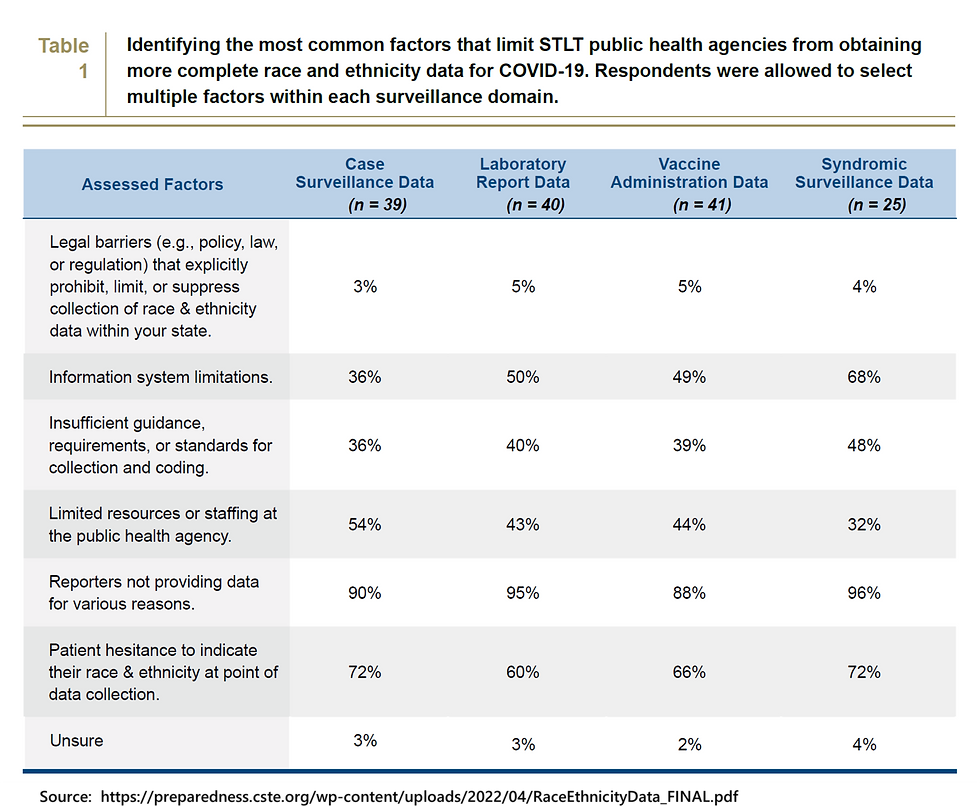
A Simple Method for Imputing Race/Ethnicity for Aggregate COVID-19 Case Counts
A flowchart outlining my imputation method, with real-world data for the state of California, is shown in Figure 3. From January 1, 2020 to March 4, 2022, more than 2 million COVID-19 cases among children and adolescents were reported in California (n=2,042,998). More than one-quarter of these cases (27.3%) were missing race/ethnicity information. The distribution of reported race/ethnicity for these 2 million cases is shown in Figure 4. The Hispanic group includes people of all races, and each of the race groups includes only non-Hispanics. The Indigenous group includes American Indians, Alaska Natives, Native Hawaiians, and other Pacific Islanders.
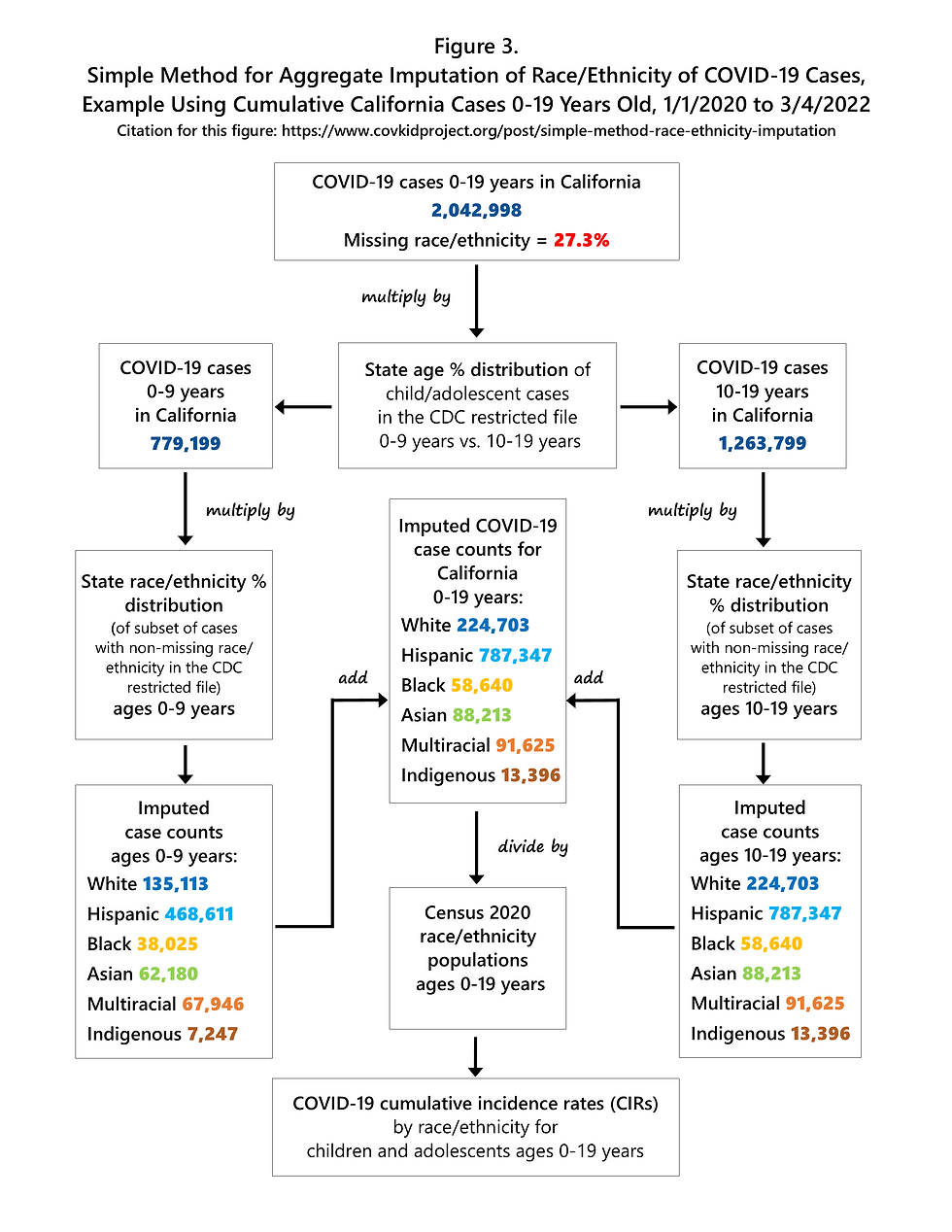
The simple imputation method explained here uses the age-specific (i.e. 0-9 years and 10-19 years) racial/ethnic distribution of cases in the state of California after subtracting out the cases with missing race/ethnicity, and then applies that distribution (i.e. percent Hispanic, percent Asian, etc.) to the total number of cases in each respective age group (Figure 3). Age-specific data are used for imputation because the race/ethnicity of COVID-19 cases varies by age group, although the magnitude of differences varies by state. After aggregate imputation, the number of cases and the percent of total cases increases for each racial/ethnic group (Figure 5), but the relative size of each racial/ethnic group to the other groups remains the same. After imputation, Hispanics accounted for 62.3% of all child and adolescent COVID-19 cases in the state of California (Figure 5).
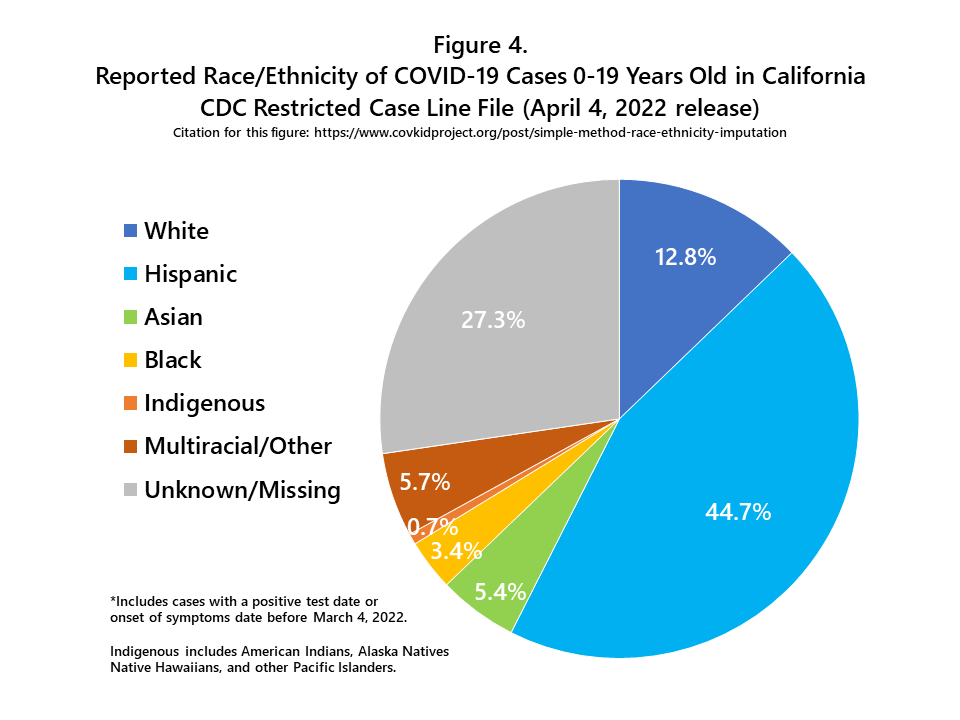
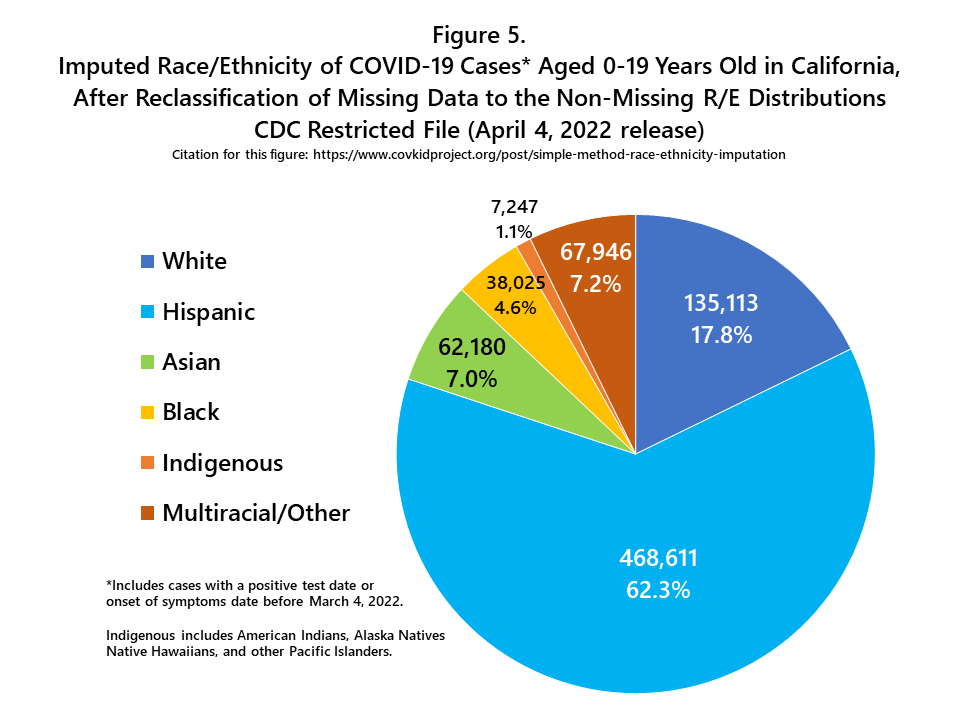
Aggregate imputation of race/ethnicity makes a substantial impact on the magnitude of cumulative incidence rates, as shown in Figure 6. A simple formula expresses the relationship between each corrected CIR (using imputed data) and its corresponding uncorrected CIR.
1 / (1 – percent missing) = ratio of corrected to uncorrected
1 / (1 – 0.273) = 1.376 (California example)

Discussion and Limitations
This method for aggregate imputation of race/ethnicity assumes that, at the individual case/patient level, race/ethnicity is missing at random (MAR), but not missing completely at random (MCAR). There are multiple systematic (e.g. logistical, organizational, and/or temporal) factors that result in missing race/ethnicity information for individual COVID-19 cases. However, I assume that these factors are not directly related to the race/ethnicity of any individual person with COVID-19, so the data are not “missing not at random” (MNAR). These statistical assumptions are based in part on empirical findings from the report Addressing Gaps in Public Health Reporting of Race and Ethnicity Data for COVID-19: Findings & Recommendations Among 45 State & Local Health Departments released by the Council of State and Territorial Epidemiologists in 2022.
Nonetheless, the assumption that race/ethnicity data missing from COVID-19 case reports are missing at random could be incorrect for one or more states. Many state epidemiologists reported in the spring of 2021 that they believed that patient hesitancy to reveal race/ethnicity information at the time of COVID-19 testing or clinical encounter was contributing to the problem of missing data. If individual hesitancy is a larger issue for some racial/ethnic groups compared with others, then data “missing not at random” (MNAR) could occur. The specific reasons for data missingness are state-specific (and probably locally specific) and are mostly unknown at the present time. Furthermore, there may be a mixture of reasons, some of which result in data MAR and some of which lead to data MNAR – meaning that the race/ethnicity of individual persons directly impacts the likelihood of that data being recorded and eventually reported to the CDC.
One possible MNAR scenario should be investigated further. If the failure of some laboratories to collect and report individual demographic data is non-random geographically, then MNAR race/ethnicity data could result, even if there is no difference in the way groups of individuals are treated within those laboratories. The reason is that in most states, there is considerable spatial segregation of household residence by race/ethnicity. So, for example, if laboratories serving predominantly Black residential areas are less likely to report COVID-19 case demographics than laboratories serving predominantly non-Black residential areas, then differential non-ascertainment of Black COVID-19 cases will result.
Another important caveat to this imputation method is less obvious, and has to do with secular trends in COVID-19 incidence. In each state, over time, the racial/ethnic distribution of cases has changed. In the California example, aggregate race/ethnicity counts were imputed only for cumulative incidence data. To apply this method for investigation of racial/ethnic disparities over time, one would need to ascertain the racial/ethnic distribution of cases at multiple points in time (e.g. monthly or quarterly) and then apply time period appropriate percentages to calculate time period specific case counts.
Conclusions
More than two and a half years into the COVID-19 pandemic, basic surveillance of new cases in the United States still suffers from serious data limitations, particularly for reporting of race/ethnicity. Evaluating racial/ethnic disparities requires complete age-specific COVID-19 case counts, so that age-adjusted (or age-specific) incidence rates can be calculated. Comparison of crude (all ages but not age-adjusted) rates is scientifically inappropriate when racial/ethnic groups have different population age distributions, as elaborated in my recent blog post on COVID-19 mortality disparities.
The aggregate race/ethnicity imputation method explained here, while not perfect, provides an immediate and accessible solution for public health professionals seeking to monitor racial/ethnic disparities in COVID-19 incidence.
Comentários